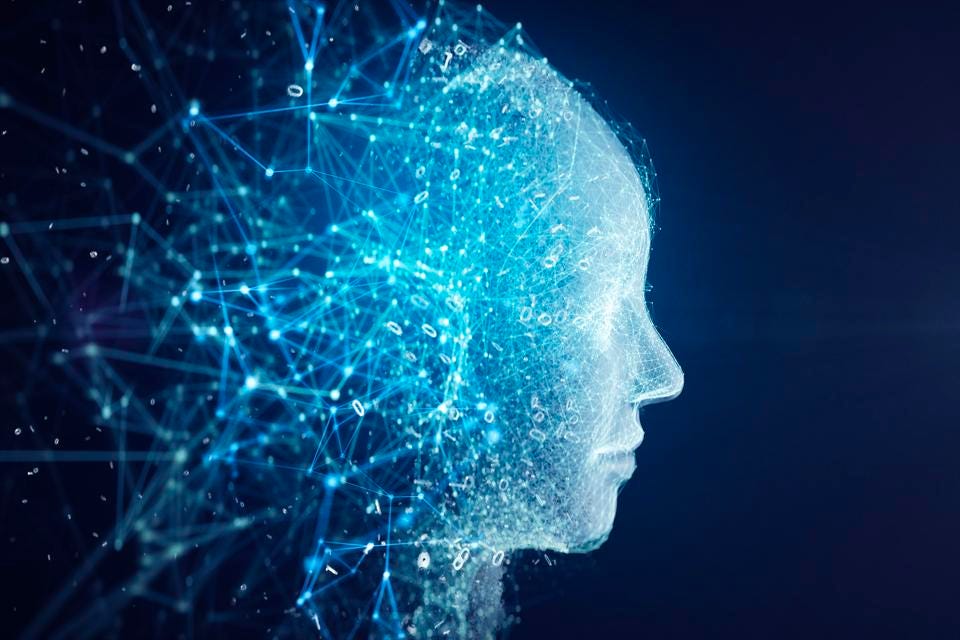
Although you may be tempted to search for specific words or phrases, machine learning can do more than simply find relevant articles. Machine learning can search documents by using fuzzy methods and topic modelling. As the field continues to evolve, this will only improve efficiency for everyone. Read on to learn more about the various methods available for machine learning. We'll be discussing some of the best here.
Unsupervised learning
Unsupervised learning is an algorithm for learning patterns from untagged data in machine learning. This algorithm is similar in that it uses mimicry as a mode of learning to create a compact internal representation. This algorithm can create imaginative content. This approach, unlike supervised learning, requires less data. Unsupervised learning is not needed to train a computer. Unsupervised learning is more useful for training machines to produce imaginative content.
An example of machine learning is to learn how to classify fruits and veggies by analyzing similarities between images. To train a supervised machine learning algorithm, it needs to be trained on a labeled dataset. Unsupervised learning is a method where the algorithm uses raw data to discover patterns that are unique for each picture. After it has learned to classify images, the algorithm can refine its prediction of the outcome of unseen data.
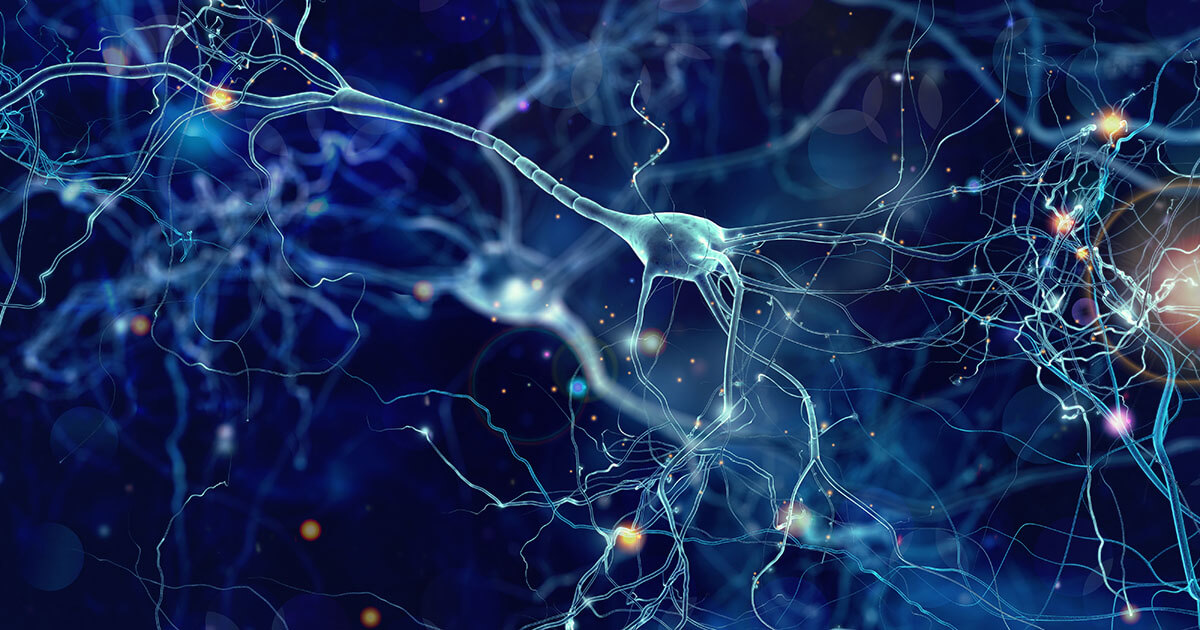
Supervised learning
Among the many types of machine learning, supervised learning is the most common. This type of learning uses structured data and a number of input variables to predict an outcome value. Supervised machinelearning can be broken down into two categories: classification or regression. Regression uses categorical data to make predictions while the former uses numerical variables to predict future value. Both of these types can be used to build models for different problems.
The first step in supervised machine-learning is to determine the type of data that will be used for the training dataset. These datasets must be collected and labeled. After the training data is completed, it is divided in two parts: the validation dataset (test dataset) and the dataset (validation dataset). The test dataset serves to validate, refine and adjust the training model's hyperparameters. The training dataset should contain sufficient information to train a model. To validate the training data and to verify its accuracy, it will be used as a validation dataset.
Neural networks
The use of neural networks in biomedicine is just one example. Recent studies have shown that deep learning can be used to assist in protein structure prediction, gene regulation, and protein classification. Metagenomics, which can predict suicide risk, and hospital readmissions, are just a few of the other applications. Interest in biomedical science has increased due to the increasing popularity of neural networks. There have been many new models that have been tested.
The training process involves setting weights for each neuron of the network. The model's input data is used to compute the weights. Training does not alter weights. This allows neural networks and their learned patterns to become convergent. However, they only remain stable in a certain state. It is necessary to have a solid understanding of linear algebra in order to use neural networks in machine-learning. You also need to be willing to spend considerable time on the task.
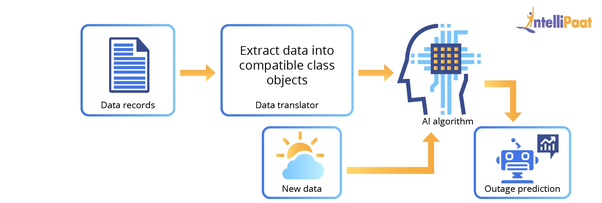
Deep learning
Machine learning algorithms often break down data and combine them to produce a result. Deep learning systems, on the other hand, look at all aspects of the problem and try to find the best solution. This is advantageous since a machine-learning algorithm has to identify objects in two steps, while deep learning programs can do this in just one. Below, we will look at how deep learning works and how it can help you improve your business.
CNNs, for example, can significantly improve vision benchmark records by max pooling them on a GPU. Similar systems were also winners of the 2012 ICPR contest involving large-sized medical images and MICCAI Grand Challenge. Deep learning also has applications beyond vision. Deep learning algorithms can help improve breast cancer detection apps and forecast personalized medicine using biobank data. The healthcare industry is being transformed by deep learning in machine-learning.
FAQ
Is Alexa an AI?
Yes. But not quite yet.
Amazon developed Alexa, which is a cloud-based voice and messaging service. It allows users to communicate with their devices via voice.
The Echo smart speaker first introduced Alexa's technology. However, since then, other companies have used similar technologies to create their own versions of Alexa.
These include Google Home as well as Apple's Siri and Microsoft Cortana.
Which countries are currently leading the AI market, and why?
China is the world's largest Artificial Intelligence market, with over $2 billion in revenue in 2018. China's AI industry includes Baidu and Tencent Holdings Ltd. Tencent Holdings Ltd., Baidu Group Holding Ltd., Baidu Technology Inc., Huawei Technologies Co. Ltd. & Huawei Technologies Inc.
China's government invests heavily in AI development. The Chinese government has created several research centers devoted to improving AI capabilities. These centers include the National Laboratory of Pattern Recognition and State Key Lab of Virtual Reality Technology and Systems.
Some of the largest companies in China include Baidu, Tencent and Tencent. All these companies are active in developing their own AI strategies.
India is another country that has made significant progress in developing AI and related technology. The government of India is currently focusing on the development of an AI ecosystem.
From where did AI develop?
Artificial intelligence began in 1950 when Alan Turing suggested a test for intelligent machines. He stated that a machine should be able to fool an individual into believing it is talking with another person.
John McCarthy later took up the idea and wrote an essay titled "Can Machines Think?" John McCarthy published an essay entitled "Can Machines Think?" in 1956. He described the problems facing AI researchers in this book and suggested possible solutions.
What does AI mean for the workplace?
It will change the way we work. We will be able automate repetitive jobs, allowing employees to focus on higher-value tasks.
It will help improve customer service as well as assist businesses in delivering better products.
It will allow us future trends to be predicted and offer opportunities.
It will give organizations a competitive edge over their competition.
Companies that fail AI adoption will be left behind.
Who is the inventor of AI?
Alan Turing
Turing was born in 1912. His father, a clergyman, was his mother, a nurse. At school, he excelled at mathematics but became depressed after being rejected by Cambridge University. He started playing chess and won numerous tournaments. After World War II, he was employed at Bletchley Park in Britain, where he cracked German codes.
He died in 1954.
John McCarthy
McCarthy was born in 1928. Before joining MIT, he studied maths at Princeton University. There, he created the LISP programming languages. In 1957, he had established the foundations of modern AI.
He died in 2011.
Statistics
- In 2019, AI adoption among large companies increased by 47% compared to 2018, according to the latest Artificial IntelligenceIndex report. (marsner.com)
- The company's AI team trained an image recognition model to 85 percent accuracy using billions of public Instagram photos tagged with hashtags. (builtin.com)
- While all of it is still what seems like a far way off, the future of this technology presents a Catch-22, able to solve the world's problems and likely to power all the A.I. systems on earth, but also incredibly dangerous in the wrong hands. (forbes.com)
- According to the company's website, more than 800 financial firms use AlphaSense, including some Fortune 500 corporations. (builtin.com)
- That's as many of us that have been in that AI space would say, it's about 70 or 80 percent of the work. (finra.org)
External Links
How To
How to set Cortana's daily briefing up
Cortana can be used as a digital assistant in Windows 10. It helps users quickly find information, get answers and complete tasks across all their devices.
A daily briefing can be set up to help you make your life easier and provide useful information at all times. The information can include news, weather forecasts or stock prices. Traffic reports and reminders are all acceptable. You can choose the information you wish and how often.
To access Cortana, press Win + I and select "Cortana." Select "Daily briefings" under "Settings," then scroll down until you see the option to enable or disable the daily briefing feature.
If you've already enabled daily briefing, here are some ways to modify it.
1. Open Cortana.
2. Scroll down to the "My Day" section.
3. Click the arrow to the right of "Customize My Day".
4. Choose which type of information you want to receive each day.
5. You can change the frequency of updates.
6. Add or remove items to your list.
7. You can save the changes.
8. Close the app